This website uses cookies so that we can provide you with the best user experience possible. Cookie information is stored in your browser and performs functions such as recognising you when you return to our website and helping our team to understand which sections of the website you find most interesting and useful.
The Insufficiency of Credit Data in a Non-Financial Crisis
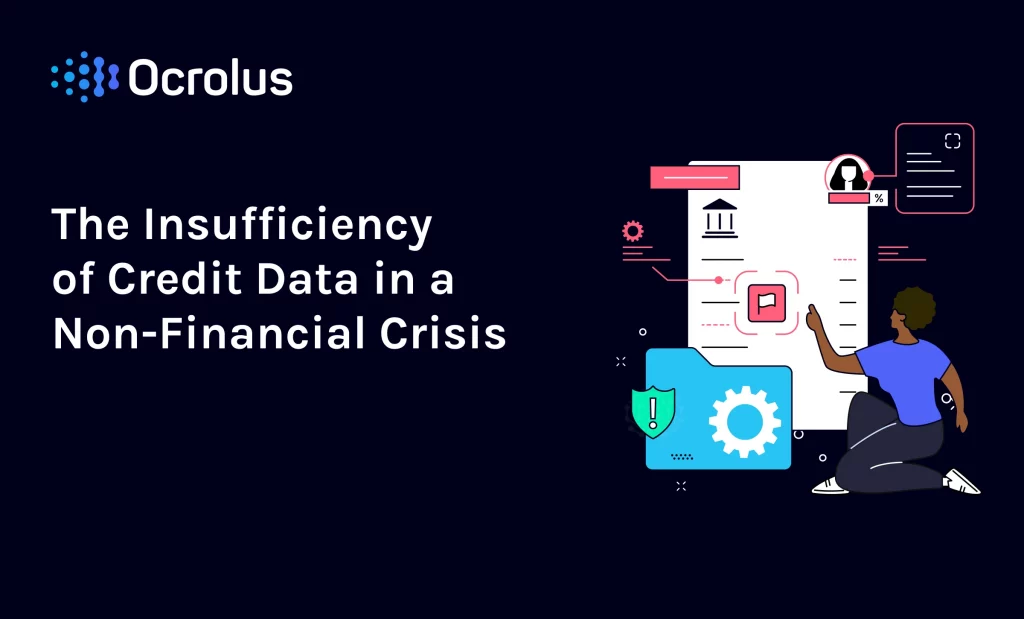
Credit scores and the data that underlie them have become a mainstay of American life over the past few decades. Consumers seeking credit – a credit card, mortgage or auto loan – and businesses seeking capital – a loan, line of credit, equipment lease, receivables advance, or commercial mortgage – have become keenly aware of their scores. Lenders of all stripes, from the oldest banks to the newest fintech companies, use credit data and scores to make approval decisions, set rates, assign lines of credit, and manage customer exposure. In most environments, this method works reasonably well. Detailed data on whether someone has paid back prior debt is generally predictive of whether he/she will in the future. However, as the recent crisis has shown us, there are times when negative external forces are so great that the paradigm shifts completely, where credit data goes from highly valuable to woefully insufficient.
In this post, I intend to cover how credit data and scores work in typical practice, how lenders tend to tweak these methods in turbulent times, and why credit data is insufficient for today’s situation. Finally, I’ll share some ideas on the necessity and potential of highly-specific, individual-level understanding of cash-flow and borrower dynamics that will become necessary in a post-covid world.
Credit = Trust
Credit is the finance-world manifestation of the concept of trust. If you have “good credit”, institutions will trust you to borrow money and to pay it back. If you have “bad credit”, they may only lend you money at a higher interest rate, or not at all. The “price” of credit is the interest rate at which it is given. While this is a slight oversimplification – rates are also influenced by macro policy, capital market dynamics, borrower market dynamics, and competition – interest rate generally correlates positively with the perceived risk of the borrower. This is true for consumer and commercial borrowers alike, from an individual borrowing $20K to buy a car to a corporation financing a $1B capital expenditure.
When borrowers apply for, obtain, pay back, or default on loans, lenders report this data to credit bureaus who match, aggregate, and make this data available to other lenders that have “permissible purpose” as defined by the Fair Credit Reporting Act (FCRA). The main bureaus in the United States are Experian, Equifax, and Transunion. There are also various specialty bureaus, including D&B, Paynet, Clarity, Teletrack, ChexSystems, and PRBC, which collect data on particular market segments. Each of these agencies collect and store borrower credit activity, a “digital reputation” of sorts that is typically of great interest to prospective lenders.
Credit Scores
A credit score is a mathematical algorithm that distills a ton of credit usage and repayment history into a single number. The most well-known and widely-used credit score is FICO, which aims to rank the creditworthiness of consumers and predict risk of default. There are also myriad lesser-known credit scores, including proprietary scores developed by particular lenders that are tuned to the risk of their specific product and borrower population. Lenders use credit scores along with many other pieces of information to approve and decline applications, set credit limits, assign interest rates, and manage outstanding customer risk.
Lending is fundamentally a business of decision-making under uncertainty and managing asymmetric risk. Lenders earn a small amount of money for each customer who repays but lose a large amount of money for each customer who defaults. Therefore, setting the right approval thresholds to manage the ratio of performing to non-performing customers is critical to maintain profitability, as is pricing the risk appropriately (i.e. setting rates and terms).
Thresholds and Risk Pricing in a Typical Challenging Economy
In a higher-risk environment, lenders can expect generally worse repayment performance from their customers. For instance, consider a lender that is able to profitably underwrite a FICO 650 customer in a benign economy. That same customer may now carry greater risk, and the lender might raise its threshold to 680. The customer also might be charged a higher interest rate to compensate for additional risk. While this “shifting thresholds” approach might be appropriate in most periods of financial stress, it is completely insufficient in an economic disruption caused by a non-financial event.
Credit Data’s Present Insufficiency
With COVID-19, we are currently experiencing a global economic crisis unlike any seen in recent memory. In contrast to the Great Recession of 2008-09, this is not a financial crisis as much as a health and humanitarian crisis that has deep financial implications. In a typical recession, spending and investment decrease, unemployment rises, and asset values go down. While these factors increase credit risk across the board, the ranking of risk with respect to previous repayment history is generally maintained. This time is entirely different. To avoid the devastating health effects of a global pandemic, people are staying home, events are cancelled, and businesses are shuttered. The impact on small businesses is perhaps greatest, where in some places, many are legally not allowed to operate.
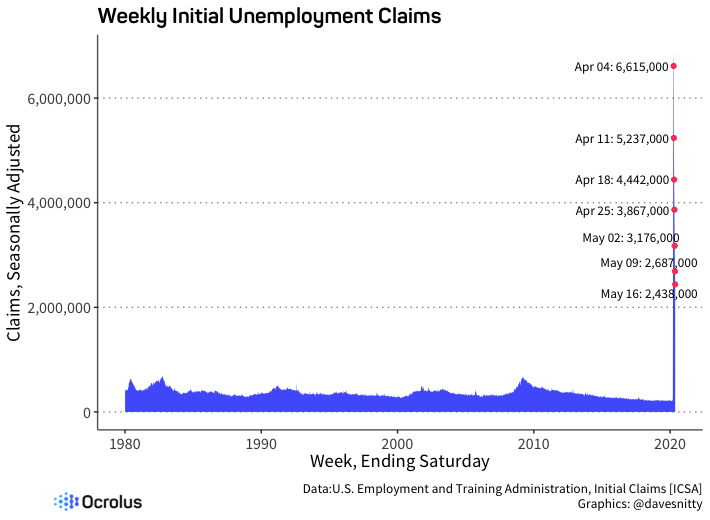
Weekly Initial Unemployment Claims Chart
Imagine two restaurant owners: one has a FICO of 625, with a spotty record of repayment and a history of being overextended; the other has a FICO of 810 and has not missed a loan or credit card payment in the past 10 years. At most times, these people would represent vastly different risk profiles, and their dramatically different histories would lead to significant differences in both access to and price of credit. Right now though, who cares? If both restaurants are closed and not bringing in any revenue, they are likely to exhibit similarly bad loan performance. The credit score is completely useless, and repayment history is meaningless if a business is legally not allowed to operate and make money.
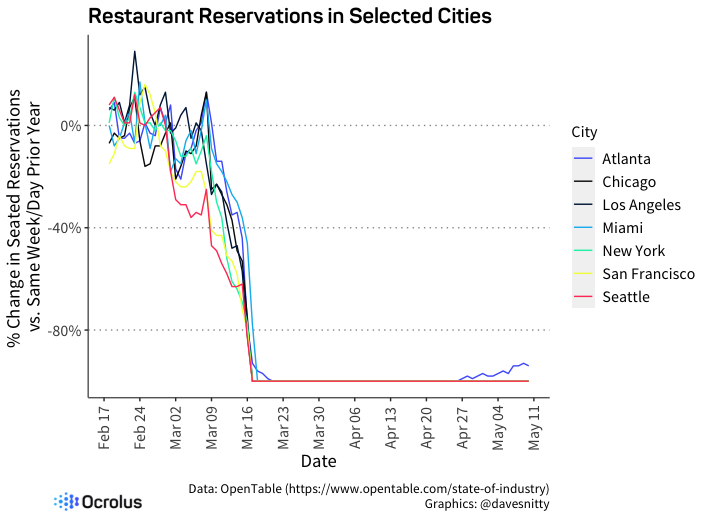
Restaurant Reservations in Selected Cities Chart
In today’s environment, performance for these entities is much more likely to correlate with information not factored into a typical credit score. Does the business have sufficient cash to cover expenses? Does the restaurant offer take-out or drive-through? Which expenses are fixed vs. variable? How much does it spend on rent? What is its monthly payroll?
What Comes After Credit?
The present crisis demonstrates and amplifies the need for highly-specific, borrower-level understanding of cash flow in making lending decisions and managing risk. While some forward-thinking lenders have begun underwriting based on cash flow, with demonstrably positive results, we are only in the early stages of this transformation. Much of the necessary and useful data remains locked in proprietary systems, complex documents, and non-standard formats. There is tremendous opportunity to utilize a wider variety of cash flow analytics and data, with greater availability, more automation, machine-accessible interfaces, higher reliability, and useful standardization. This is true for consumer and business data alike.
Financial Data
An incredible amount of information resides in a person or company’s bank accounts. Current and historical bank balances provide valuable insight into liquidity, while transaction-level records can yield vast insights if closely analyzed. Understanding the date, merchant name, transaction description, and size of every bank or credit card transaction a customer has made can produce a much deeper picture of a borrower than credit bureau data alone. With such information, lenders can understand the seasonality of a business’ cash flow and forecast future revenue. They can break down which expenses tend to be recurring vs. episodic to build a picture of future obligations. They can understand the sources, size, and consistency of a person’s income. Having this detailed picture of daily cash flows is advantageous in building a resilient risk evaluation strategy.
Of course, the reality of accessing, processing, and extracting useful analytical data from financial records is incredibly complex. There are several thousand banks in the United States, each with its own data schema and document format. Real-world data is also notoriously messy, filled with anomalies, omissions, and inconsistencies. Even if a lender is able to procure the data reliably – a challenge in and of itself – transforming it into useful information with meaningful value is a tall order. Some lenders have large teams of data scientists, engineers, and analysts working on exactly these problems, and their jobs are not remotely easy. Data aggregators like Plaid help lenders gain programmatic access to bank account data. Ocrolus helps lenders merge electronic bank data, complex data derived from documents/statements, and other information into a single, standardized API while also providing a layer of analytical metrics and decision-ready insights.
Operational Data
For businesses, valuable data also resides in accounting systems, payment processing systems, invoicing software, and inventory management systems. For consumers, data from employers’ payroll systems can provide useful confirmation of income and employment, and data from gig economy platforms can offer insight into the consistency of, for instance, a Lyft driver’s earnings. Given the vast constellation of system types and vendors, accessing and making sense of this data is highly complex. Which data points are valuable and which insights are relevant are quite specific to the financing product being offered. For instance, a lender offering business financing that is secured by receivables would be well served to understand the data in its borrowers’ inventory management systems. Again, these efforts are likely to involve a broad and complex set of data and will benefit from the right blend of automation and machine learning with subject matter expertise and human-in-the-loop decisioning.
Ambient Data
Depending on the prospective customer and the particular financial product being offered, there exist innumerable sources of information that might be relevant to a lending decision. This can be considered ‘ambient data’. While the financial and operational data sources are “customer permissioned”, ambient data is quite different; aggregating and contextualizing it presents its own set of challenges and opportunities. For instance, if a lender is considering a loan to a barbershop, there are many pieces of information that could be of theoretical value. Could one parse the sentiment, rating, and timing of all online reviews? Is it possible to use cellular data to measure foot traffic? Is it possible to compare its menu of services to every similar barbershop and rank it in terms of breadth of offering and price competitiveness?
In theory, all this information exists somewhere, and there’s a reasonable chance it correlates with outcomes a lender desires to predict. However, a multitude of considerations accompany this hyper-ambient approach: Is it worth the money a lender would need to invest? The effort? The complexity of implementation? The fair lending and privacy implications? How significant does the ‘lift’ need to be, and at what scale of lender, for the investment to pay off?
These approaches make brilliant powerpoints and compelling conference talks, but while they might be utilized today in very specific cases, they have not been widely adopted. For broad and aggressive use of ambient data to break into the mainstream, we likely need to see an inflection point in machine learning technology, and likely in regulatory policy as well.
Future Implications
The crisis caused by COVID-19 has touched every part of society, and its effects will be felt for years – if not decades – to come. The best lenders will awaken to this new reality and develop strategies that are powered by a broader and richer set of borrower-specific data, aided by technological advances in machine learning, automation, and human-in-the-loop decisioning. Ultimately, adopting this more data-driven approach will increase access to credit for consumers and businesses, leading to more economic activity and prosperity.