This website uses cookies so that we can provide you with the best user experience possible. Cookie information is stored in your browser and performs functions such as recognising you when you return to our website and helping our team to understand which sections of the website you find most interesting and useful.
Use Automation to Filter Customers Early in the Lending Process
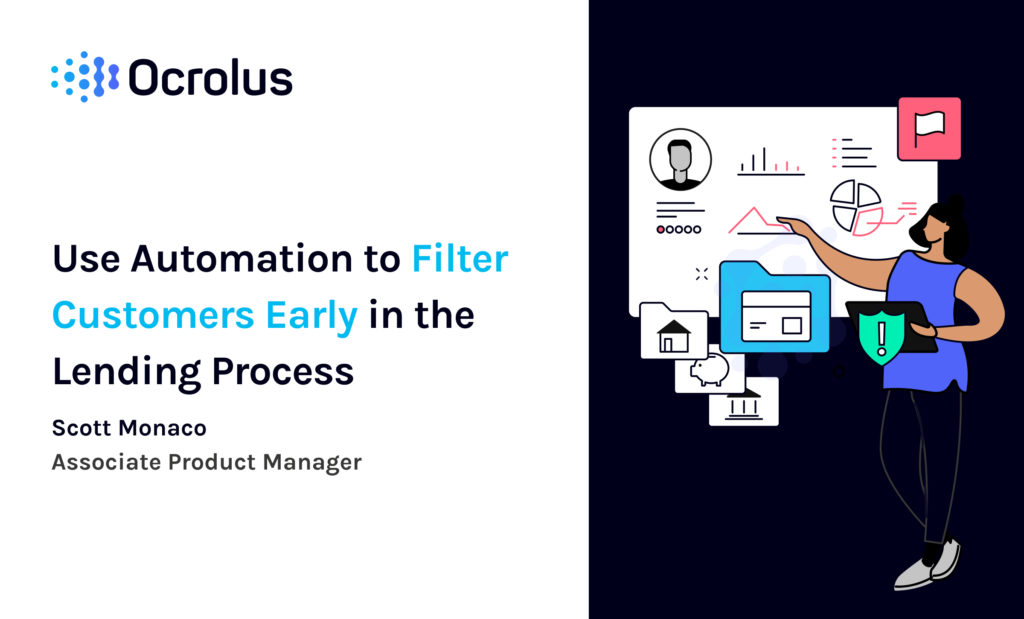
The number of borrowers falling behind on credit card payments and personal loan payments is rising more quickly than usual. In March 2022, delinquencies on subprime credit cards and personal loans rose for the eighth month in a row, according to The Wall Street Journal.
Mortgage lending has always involved risk, but in the current environment, many borrowers have become even riskier for lenders. Rather than wasting time and energy on loan applications with a remote chance for success, consider using automation to help filter out unqualified customers earlier in your lending process.
Misaligned customers can be expensive
Making loans to bad customers who will make late payments or eventually default is clearly expensive. It is also costly to invest significant staff time on a prospective borrower that has little chance of qualifying for a mortgage. Losing a customer, even through default, means you’ve lost the future revenue expected from that account and the time and expense it took your staff to acquire that customer. Additionally, even if a customer’s failure to pay his obligations is his own fault, he is likely to leave with a negative view —and to share that negative view with other potential customers— damaging your long-term revenue.
Doing business with a customer who is a bad fit for your company or product costs time and money, as well as the opportunity cost of spending that time on good-fit customers. Currently, delinquency rates on loans are on a slight upswing. To avoid expensive problems down the road and maintain a profitable business, you need to develop risk assessment methodologies that address new and emerging customer bases, like those new to credit. This work, combined with automation tools to weed out customers who present a high risk of default, enables lenders to increase origination rates while maintaining the same level of enterprise-level credit risk.
Bad news early is good news
Your first step is to break up with bad customers before they have the chance to default on loans and injure the bottom line. Gartner research predicts that by 2025, three-quarters of companies will ditch customers who aren’t a good fit for them, as the cost of retaining poor-fit customers is higher than the cost of acquiring customers that are a good fit. Rather than waiting for a customer to leave, companies are getting more proactive and ending the relationships on their own terms.
However, in the mortgage lending process, it can be difficult to recognize a borrower who may become a poor fit. That’s because the mortgage industry continues to largely use manual processes and rely on old validation methods to determine creditworthiness, such as credit scores. Further, a greater segment of the population is deriving its income from non-traditional sources, making it difficult to assess the creditworthiness of a borrower using legacy underwriting processes. While credit scores track people’s bill-paying history, they don’t take into account an individual’s income or savings, which can be stronger predictors of mortgage risk. Cash flow analysis derived from transaction-level bank data, paired with newer automated tools, can help mortgage lenders see a more complete picture of a borrower’s financial situation rather than simply relying on one metric.
Automation and analytics can better assess client fit
By automating the mortgage application process, lenders can speed up workflows and review more borrowers’ files in much less time. Instead of a long wait to get approval, applicants can get faster answers.
In addition, a robust automated process can improve access to cash flow analytics to give lenders a more holistic view of a potential borrower’s financial health, which can help lenders identify a good (or bad) fit customer earlier in the process.
For example, with Ocrolus, lenders can submit a potential borrower’s banking information through bank data feeds, PDFs, or cell phone images. With document capture technology, Ocrolus can transform unstructured information into clean, structured data—allowing lenders to make sense of cash flow data from multiple sources with one unified view.
The software normalizes captured data, enabling the lender to perform detailed income analysis and risk modeling. Reliable, detailed bank data can provide a much deeper picture of a potential borrower’s financial status than credit bureau data alone. Through income analysis, lenders can factor in non-traditional income, assess debt capacity and identify recurring transactions. In addition, a lender’s risk models based on monthly income may help that lender predict the risk of default more reliably and quickly than traditional models.
Lenders can filter out bad customers while also capturing new customers not serviced by traditional lenders relying on credit-bureau data alone through the use of automation and cash flow analysis before underwriting. This upfront work may reduce the expense of unqualified borrowers, expand the top-of-the-funnel for acceptable credit risks and work to maintain—and gain—profitability by working with qualified borrowers.
If you’re interested in understanding how Ocrolus can help lenders better understand a borrower’s cash flow data, try out a free demo today.