This website uses cookies so that we can provide you with the best user experience possible. Cookie information is stored in your browser and performs functions such as recognising you when you return to our website and helping our team to understand which sections of the website you find most interesting and useful.
How training data drives AI for complete, accurate document automation
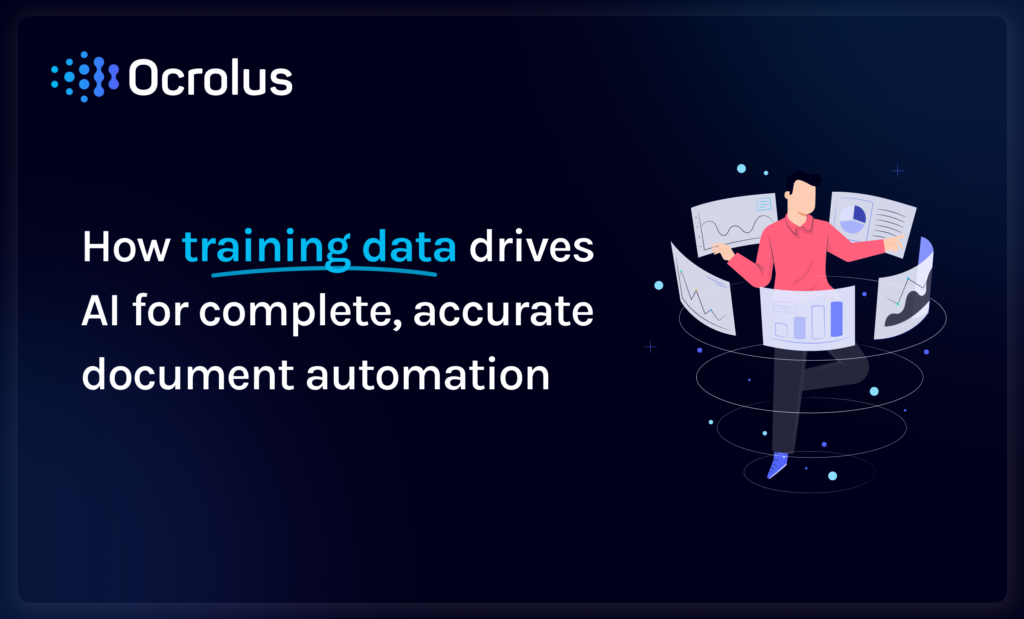
The rapid advancement of AI and machine learning is driving significant transformation across many industries, and lenders and financial institutions are uniquely poised to benefit from their strategic application.
With large amounts of data and complex decision-making processes, lenders can use AI to automate and streamline their operations, improve efficiency and accuracy and reduce fraud.
In McKinsey’s Global AI Survey report, nearly 60% of respondents in the financial services sector reported their companies had embedded at least one AI capability. The most common application of these technologies includes process automation for structured operational tasks and machine learning to detect fraud and support underwriting and risk management.
But in financial services, there is zero tolerance for AI-generated errors. High-stakes decisions, such as a $100k small business loan, require accuracy. This requires precise, high-quality training data to ensure AI can classify, capture and analyze data correctly.
Complete and accurate training data is particularly crucial for financial institutions that rely on AI to process loan documents. Training data significantly affects the quality of the document processing algorithms, which, in turn, affects the institution’s risk assessments and lending decisions.
Why is high-quality training data useful for AI?
AI-driven lending analysis can only be reliable if the technology provides high-quality data to feed the machine learning process.
These technologies rely on training data to learn and classify patterns and relationships accurately. Without quality-labeled training data, machine learning and AI models will perform poorly, leading to inaccurate predictions and poor performance.
For example, let’s say a financial institution wants to automate its loan document processing using AI. The AI model is trained on a dataset of loan documents, along with their classifications and extracted data. If the training data contains errors, inaccuracies, or inconsistencies in the labels, the AI model will learn from these errors, leading to incorrect predictions and analysis.
Without accurate training data, AI models can continue to use this incorrect pattern, leading to increased risk exposure for the financial institution. Because of this mislabeled data, lenders risk approving higher-risk loans, resulting in higher default rates and potentially huge financial losses.
Similarly, if the training data contains biases, such as underrepresenting certain demographic groups or geographic regions, the AI model will learn these biases, potentially leading to discriminatory lending practices. This can result in legal and reputational risks for the financial institution.
For reasons like these, it is crucial for financial institutions to ensure the accuracy and fairness of the data used in their decision-making process – particularly when using AI and machine learning models to automate lending decisions.
On the other hand, by implementing technologies that focus on ensuring data integrity, lenders and financial institutions can avoid unnecessary defaults or unforeseen risks.
How does artificial intelligence improve the document automation process?
As more financial institutions implement AI-driven technologies for lending decisions, complete and accurate training data plays a vital role in their success. By investing in technologies that focus on data preparation, labeling and quality, lenders can identify potential risks and mitigate them before they become a problem.
By leveraging AI to automate document processing, financial institutions eliminate manual review and “stare and compare” work such as loan processing, identity verification and cash flow lending analysis.
By automating lending decisions, lenders can significantly improve efficiency, speed up loan processing time, and minimize human error. For example, an AI-powered loan processing system can analyze thousands of loan applications within seconds, a task that would take several hours for a human to complete. This not only saves time but also reduces the risk of human error and inconsistencies in lending decisions.
Ocrolus’ massive and continuously growing training data set now contains more than 240 million pages of financial documents”
How Ocrolus Uses Training Data for AI to Advance Our Complete Document Automation Solution
While the technology has advanced rapidly in recent years, unassisted state-of-the-art AI models aren’t perfect yet.
This is where Ocrolus’ completely in-house data labeling capability is a distinct advantage. While most firms must purchase training data for AI, Ocrolus is able to mint our own training data as a byproduct of doing business.
Today, Ocrolus processes the majority of documents without any human review. For new document types or lower-image-quality scans that cannot rely purely on automation, we incorporate a human-in-the-loop process to train and improve our models continually. Our own employees verify information, supported by algorithmic quality control to ensure we return perfect data to our customers to support their lending decisions. This data then powers a feedback loop that continually trains our models to become smarter and more complete, reducing the need for human review in future cases.
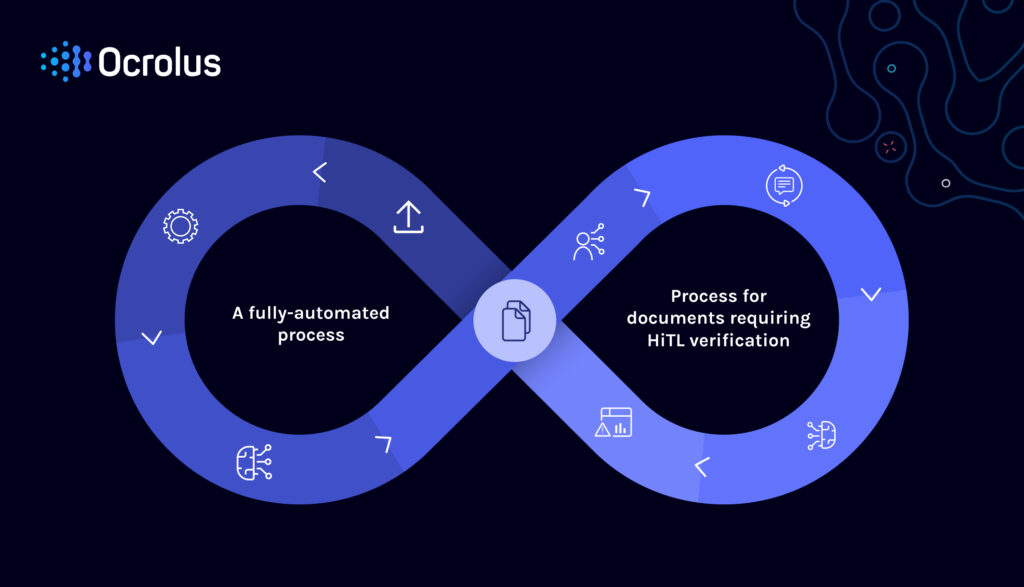
Simply put: The agility of Ocrolus’ human-in-the-loop processes means Ocrolus can say “YES” to automating tasks for which training data doesn’t yet exist. As these tasks become more prevalent, Ocrolus AI leverages the stream of outputs as labeled data. Once sufficient training data is gathered, the end result is a highly automated capability in which humans and AI work together to achieve reliable data delivery.
Book your demo to discover how Ocrolus’ training data and advanced AI provide a complete, accurate document automation solution to power your lending processes.